AI hype has peaked so what’s next?
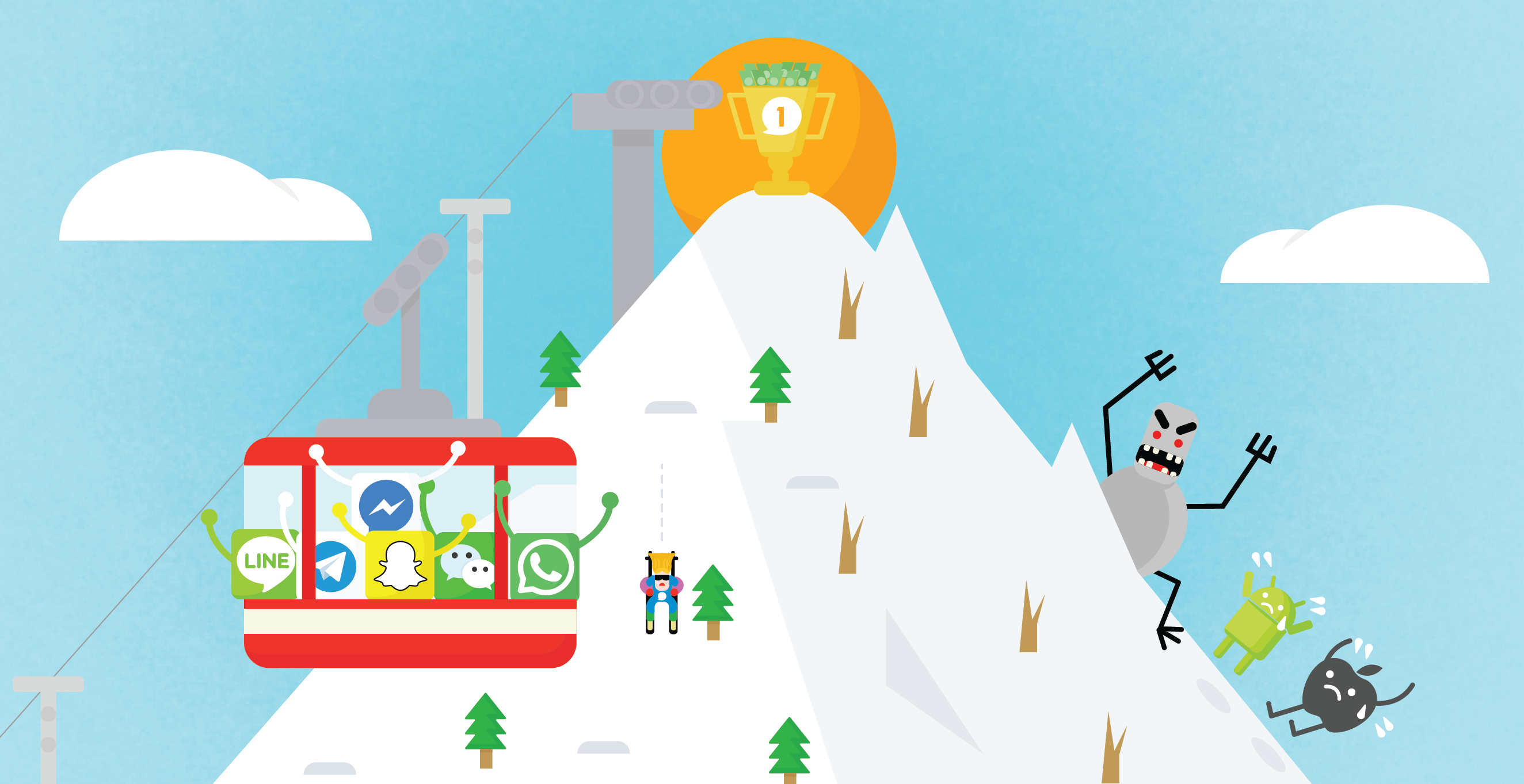
2017 has been the year of AI, reaching a fever pitch of VC and corporate investment. But, as with any hot technology, AI is outgrowing this phase of experimentation and hype. According to research firm Gartner, we’re past the “peak of inflated expectations.”
Next up is a necessary recalibration of the space—one that will separate the winning AI-driven companies from all the noise.
[AdSense-A]
This next phase of AI is all about substance over flash; the focus now is on the hard work of building and strengthening real businesses that can go the distance. Since 2012, the common exit for an AI company has been acquihire—acquired for talent or technology, not business performance—with many companies selling for below 50M.
Simultaneously, the behemoths of tech like Facebook, Google, and Amazon are innovating in AI at a rapid pace—displacing smaller competition by releasing new products or open sourcing more AI tools. In this highly competitive and rapidly evolving environment, cool tech isn’t enough. It’s essential for startups to build AI strongholds that can outlast the competition and compete with the big guys.
But, what are the prerequisites and ingredients for a winning AI-driven business? As VCs evaluating the space, we’ve come up with a few key attributes that help us identify the strongest players. I like to call these attributes “moats.” Each additional “moat” helps secure your castle—widening the gap between you and your competition while creating more value for your customers.
- Proprietary Data
As an emerging competency in the enterprise, integrating AI functionality into products is giving companies a competitive edge—at least, for now. But, AI-as-a-Service is not far off. Google, Microsoft, Amazon, and Salesforce have made outsized investments in the space. Eventually we’ll see a democratization of AI capabilities as algorithms become increasingly more streamlined, standardized, and universally available. This leaves the true value in the data itself. Massaging a volume of data for use and getting it right is no easy feat. But startups need to ask themselves what the long-term value of their data is. Is it exclusively yours or can it be easily replicated or acquired by a competitor? The ability to acquire proprietary data is a key signal to us whether or not a company will eventually find itself part of a commoditized pack or can maintain meaningful differentiation long-term.
- Team Domain Expertise
A differentiated AI solution isn’t just about having a novel algorithm and throwing unique data at it; it’s about having a team that understands what to look for in the data—someone who can turn the knobs and dials and adjust the algorithm to help an AI system learn to recognize correct and incorrect answers. Contrary to popular wisdom, this person is not necessarily an engineer or a data scientist. It’s someone who can understand actuarial tables if you’re in the insurance business. Or, if you’re in defense, it’s someone who can understand the signals that are most discriminating in detecting potential threats. These domain experts give AI teams a leg up in making their products relevant, practical, and indispensable to their target markets. This level of specialized “human tuning” sets leading AI solutions apart from simply “renting” algorithms for general data processing.
- Workflow Position
So you have unique data and you built a team with both domain and technical acumen, but have you built a system that fits within the target users’ daily or regular work flow? The best AI solutions are those that exist in what I call an “operational loop,” where they are constantly being fed new data and seeing regular user engagement. A great example of this is Gong.io, which analyzes daily sales recordings and provides performance recommendations. Not only does this type of position in the workflow increase customer stickiness—it also helps the system get smarter over time. Having a team with comprehensive domain knowledge comes into play here: is your AI system appropriately trained to ask the right questions for optimal pattern recognition and creation? Is it learning, adapting, and becoming an increasingly more essential piece of a user’s workflow? Human tuning is necessary at the outset of any AI learning process, but the system itself must develop and increase its performance for the specific customer. This ability drives inherent advantage over the static software available in the market.
- Degrees of Customer Value
What is the actual impact of your AI solution on a use case? What exactly are you doing better? Is it 10 times better or is it 100 times better than the status quo? For example: say you’re a doctor, and there’s a new piece of software that helps you analyze X-ray images a little bit more conveniently. It already filters out unnecessary visual info and zooms into the key areas where you’ll need to look.
That’s a tool, I’d say. It might help one doctor be more productive—a 2 to 5x multiplier. But if you take the same doctor and gave him a tool that went through all the images he needed to review in an afternoon and narrowed scope to only the priority images—let’s say, three out 500—that would be a “force multiplier”—a 10x value. The 100x is the most exciting, and that’s where a system could look through all the X-rays automatically, and go straight to a diagnosis. This level of near total automation requires a ton of trust, hence the value of domain experts to set it up and AI learning to capture data, patterns, and insights at scale. As such, we try to invest in companies that land in the 10x value with the promise of eventually closing in on 100x.
If you’re one of the AI entrepreneurs out there: what are your AI moats? How defensible are they? What are you building that is stronger than just technology and talent? Enterprise businesses want and need you (and I want to hear your pitch). But only the strongest AI companies will be able to outlast the pack of startups and outpace the big tech companies. Over next five years, AI will continue to expand as a layer across every enterprise business process—from sales to marketing to customer service to product development to finance to operations. How are you going to be a key player driving that transformation?
Read more: https://techcrunch.com/2017/09/30/ai-hype-has-peaked-so-whats-next/